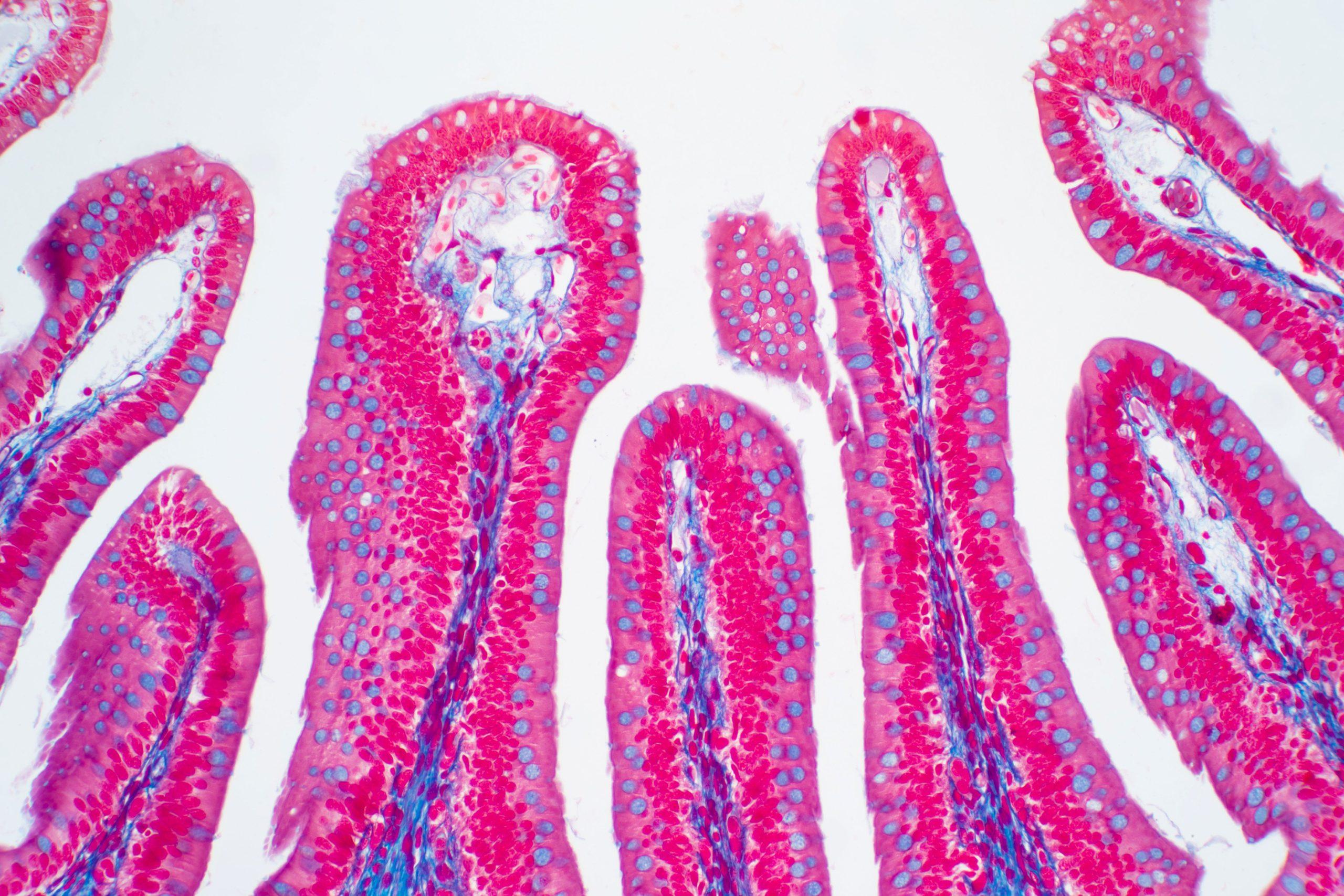
A microscopic view of the large intestine, and the many different cells that comprise it. Source: AdobeStock_316295145
By Optimizing Cell Classification, the New Tool Running on Bridges-2 Promises Better Understanding of How Individual Cells Help Organs and Tissues Function
Scientists studying the genetic activity of single cells in the body must organize them into clusters to understand how they work together to make organs and tissues function. But defining clusters whose resolution is fine enough to be informative, while at the same time reliable enough to be reproducible is a challenge. Scientists from the University of California, Berkeley; the University of Southern California; and elsewhere used PSC’s flagship Bridges-2 system to run their software, Dune. The new tool takes sets of cell clusters and optimizes them to ensure they’re biologically meaningful and useful, outperforming previous methods for defining clusters.
WHY IT’S IMPORTANT
The marriage of high-throughput DNA and RNA sequencing technologies with microscopy has opened up a tiny but promising world for doctors and life scientists. Today, scientists can measure gene activity in single cells, allowing us for the first time to understand what’s going on in the body at the scale of individual cells and tissues working together.
These advances promise to give us a vastly more complex and nuanced understanding of how the body works in health and disease, by identifying specialized types of cells within a given organ. Such clusters of cells help an organ work efficiently by splitting the work with other specialized cells in the organ. They may also be better targets for disease therapy, helping to make drugs more effective with fewer side effects.
There’s just one problem: What does it mean when one cell’s activity is different from its neighbor’s? We might think that any difference is important. But what if one cell is activating a given gene just because it’s received a chemical signal that another, otherwise identical, cell hasn’t yet? If so, the next time you measure the cells’ gene activity they might be the same, or reversed — the difference isn’t meaningful, because it isn’t reproducible.
Scientists have created a series of rules for defining cell clusters that are both as small as possible and as reproducible as possible. That way, we get the most precise understanding of how the cells in an organ are working with each other without splitting into meaningless, random differences. The rules, by and large, work, but they are extremely expensive in the amount of experts’ time they require — and experts do occasionally differ on what if anything a given cluster may mean.
“Clustering is a very old idea in statistics. It’s just finding groups of things that are similar. And it’s fundamental to most single-cell analysis, because we want to be able to make sense of what are the different cell types involved … It’s always a matter of what do we think are the right clusters? And why do we think it? You could always go a different route and basically have very similar justifications for it.”
— Kelly Street, University of Southern California
Hector Roux de Bézieux, a postdoctoral fellow from the University of California, Berkeley, and Kelly Street, an assistant professor of population and public health sciences at the University of Southern California, wanted to find out if they could use the power of high performance computing to provide an objective set of clusters for cells. They based this classification on the cells’ production of mRNA. These are the nucleic acids produced when a cell transcribes its genes from the DNA blueprint to a kind of “work order,” an RNA molecule that can direct production of proteins or other activity in the cell.
Working with their former faculty advisor, Professor Sandrine Dudoit, and colleagues at a number of institutions in the U.S. and Europe, their tool of choice for this project was PSC’s NSF-funded Bridges-2 supercomputer. The scientists got time on Bridges-2 through an allocation from ACCESS, the NSF’s network of supercomputing resources, in which PSC is a leading member.
HOW PSC HELPED
The problem came down to the need to make cell clusters, defined by differences in their single-cell transcriptome sequencing (scRNA-Seq) fingerprints, as small as possible while remaining reproducible. They designed Dune, an implementation of their strategy using the popular R statistics software package, to take as its input rough clusters of cells defined by current computer-driven clustering methods, with somewhat arbitrary definitions set by humans. Dune would then mix and match the cells in these initial clusters to break them into clusters as small as possible until further mixing wouldn’t improve the clusters.
“[Dune is] definitely computationally intensive … [it] does an exhaustive search over pairs of clusters from within a given clustering [and searches] over every possible pair of, say, 100 clusters to see which ones could be merged, and which of those merges would be most optimal. I’ve tried running similar things on my laptop a couple of times, and it does not work … I think it would have been very, very difficult to do this without these sorts of computational resources.”
— Kelly Street, University of Southern California
Bridges-2 proved an ideal place to run their software. The work required hundreds of different reshufflings of the clusters. Bridges-2’s hundreds of powerful computing nodes were able to run Dune in minutes rather than days. First using simulated data — so that the collaborators would know what the “ground truth” clusters were — Roux de Bézieux ran Dune using different input clusters of the same sets of cells.
The results were encouraging. Dune produced similar clusters regardless of how well or badly the input clusters sorted the data. It also automatically reached a stopping point at which the clusters couldn’t be improved on, again despite the quality of the input data. Best of all, the final clusters it produced agreed well with the ground truth of the simulated clusters.
Taking Dune to real scRNA-Seq data, the scientists then found that the software improved significantly on the performance of current methods, particularly those requiring more human or computer time for defining clusters. The team reported their results in the journal BMC Bioinformatics in May 2024. They’ve also made Dune available to other researchers through an open-source software package released through the Bioconductor Project and written using R.