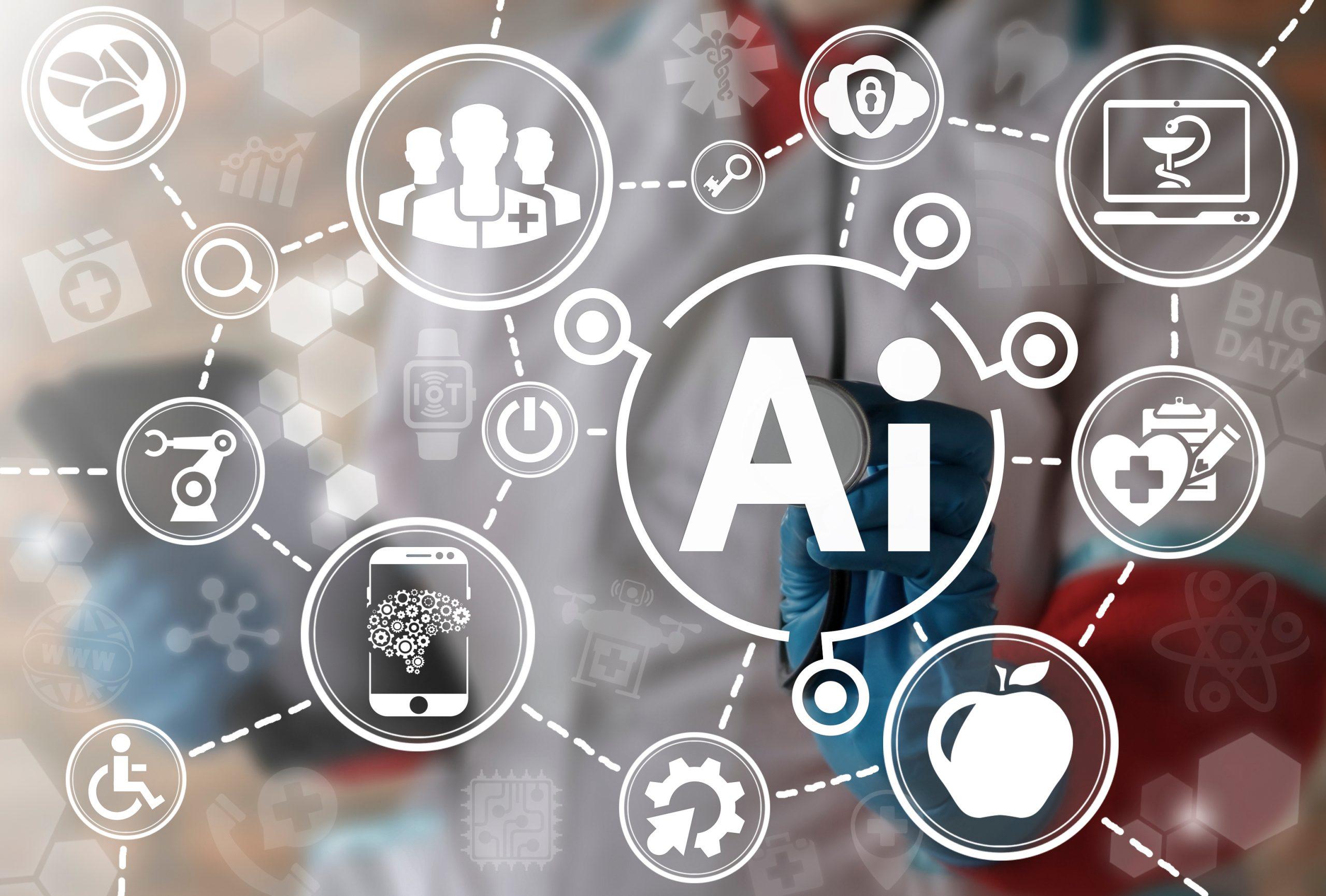
Initial Work With PSC System Pilots AI That Went On to Predict Colorectal Cancer Genetic Status That Would Otherwise Require Lengthy Lab Testing
Diagnosing cancer relies heavily on human expertise and lengthy genetic testing, which aren’t always available. A team from Harvard Medical School used PSC’s Bridges-2 supercomputer in pilot work on an artificial intelligence (AI) program that went on, from microscope images and other clinical data, to successfully predict survival in colorectal cancer patients, as well as the tumor’s genetic status without genetic testing.
WHY IT’S IMPORTANT
When it comes to diagnosing cancer, often we’re applying 21st-Century treatments to diagnoses made with hundred-year-old technology. Pathologists employ intensive training and vast experience to categorize cancers — which helps determine optimal treatment. While genetic tests are available to help predict treatment outcomes, they often take precious days to weeks to complete. They also aren’t available in many parts of the world. When that information is missing or delayed, cancer can progress and become more dangerous.
Pathologists are good at what they do. But the human eye and mind have limitations and can only do so much. Methods to automate routine pathology findings, which can vary from pathologist to pathologist, would be good. But even more useful would be tools to predict which tumors will respond well to standard treatment, and which will require more aggressive or experimental treatment. This is especially important in colorectal cancer, in which early stage tumors can vary greatly in their aggressiveness.
“[Today, pathologists] manually look at the pathology images under the microscope and based on their experience and what they have seen before … [they] define whether this is likely colorectal cancer [or] likely some other cancer types. Within colorectal cancer, they can also identify important parameters, things like whether this is high grade or low grade … So that’s the current state of the art we are using in the twenty-first century. Surprisingly, we are still using a technology that was developed around 100 years ago …”
—Kun-Hsing Yu, Harvard Medical School
Kun-Hsing Yu of Harvard Medical School and Boston’s Brigham and Women’s Hospital wondered whether an AI tool could use microscopic images and other data to improve what human experts could accomplish. To develop this AI, Yu and collaborators Jonathan Nowak at Brigham and Shuji Ogino at Harvard’s T.H. Chan School of Public Health, Brigham, and the Broad Institute of Harvard and Massachusetts Institute of Technology and their teams used a number of supercomputing resources, including PSC’s NSF-funded Bridges-2.
HOW PSC HELPED
The scientists focused on colorectal cancer, the second-largest cause of cancer deaths in the U.S. In the first stage, they trained their AI using pathology microscope images from The Cancer Genome Atlas (TCGA) that were labeled by experts to show which contained cancer cells, and if so, what their molecular profiles were according to the genomic sequencing results. Bridges-2’s massive data-handling abilities proved useful for the work because the high-resolution images contained billions of pixels, and so the computer had to juggle a lot of data as it was learning to distinguish between the different slides.
Once the AI, which they called the Multi-omics Multi-cohort Assessment (MOMA) platform, had learned to distinguish between the labeled slides, the investigators validated its performance on slides in which the “right” answers weren’t given to the system. For this validation they used other data sets, including the Prostate, Lung, Colorectal, and Ovarian (PLCO) Cancer Screening Trial cohort and the Nurses’ Health Study-Health Professionals Follow-Up Study (NHS-HPFS) cohorts, two long-term trials studying large cohorts of participants. Together, these data sets provided a huge trove of data that really put the AI to the test. In particular, the NHS-HPFS cohorts were challenging because they used smaller-sized images than the others, so the AI had less detail to work with.
The initial validation was a great success, with the AI successfully identifying the presence and molecular profiles of cancer cells. It out-performed previous AI methods for analyzing cancer slides by 7 to 29 percent. In addition, MOMA successfully split the patients into two categories, long-term survivors who did well with standard therapy, and short-term survivors who were going to need more extensive treatment. The two categories predicted by the AI have a statistically significant difference in their survival outcomes, a distinction human pathologists can’t make.
But the Harvard team wanted to do more. Using more complete information about each patient — including demographic data and other details in their overall medical records — they also trained MOMA to go one step farther. The AI made predictions about each patient’s microsatellite instability (MSI) status. This genetic signature helps identify patients whose tumors are likely to be resistant to standard therapy. Currently MSI status can only be determined by genetic tests that can take weeks to complete.
“One task [for MOMA] is about detecting the molecular diagnosis — trying to predict whether this patient has a mutation in a certain gene or not, as the [optimal] treatments may be different … That task pathologists cannot do [without genetic testing], so any progress is progress. We are quite fortunate in that not only did we make some progress but [made] what we believe is a big leap compared with what we have currently.” —Kun-Hsing Yu, Harvard Medical School
MOMA proved effective at predicting MSI status, with AUROC scores — which measure the accuracy of the prediction, with 1.0 being perfect — between 0.76 and 0.88. This is a major finding, because the AI was able to find clues in the microscopic images, not visible to humans, that helped it predict MSI without actual genetic data. Applying MOMA to patients could supply this potentially life-extending information in places where genetic testing is unavailable, or much more quickly where it is. The team reported their results in the journal Nature Communications in April 2023.
Next, the collaborators would like to improve MOMA’s performance, by testing it against more and more international data sets. Another possible avenue for improvement is incorporating an AI technique called enhanced representation learning to improve MOMA’s speed of learning. Finally, they’d like to see if giving MOMA more data to work with, including radiology imaging data, more detailed pathology data, genetic testing results, and more extensive clinical data, would improve its predictions.