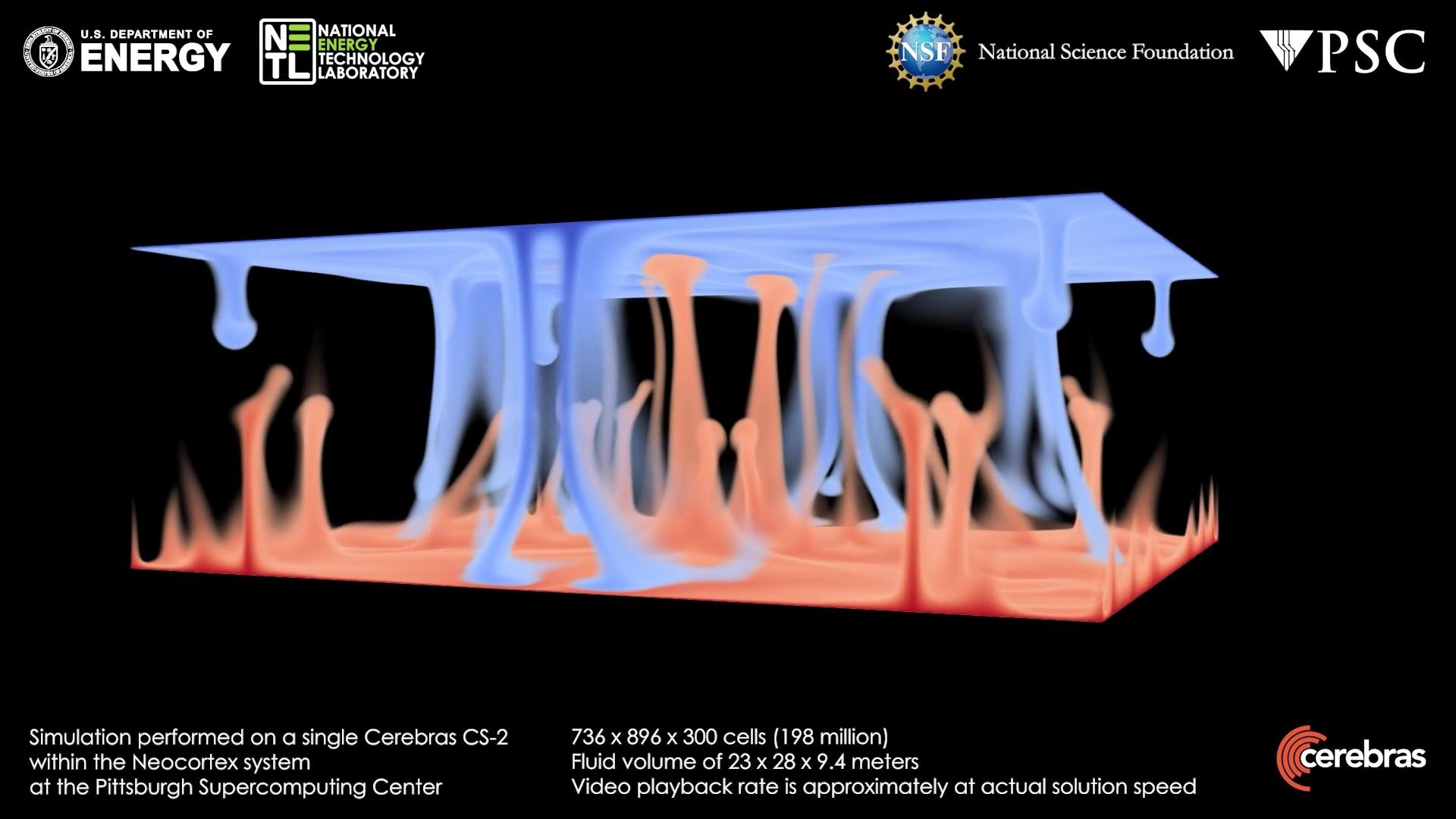
Neocortex-computed still-image from a simulation of the classic Rayleigh-Bénard convection problem, which shows how a fluid layer begins mixing as it’s heated from the bottom (red) and cooled from the top (blue) in a gravitational field.
Real-time simulations offer better, faster predictions in dozens of real-world problems
Using computational fluid dynamics, scientists can simulate the flow of a fluid — important in thousands of real-world problems — with great accuracy. But even on the fastest supercomputers it’s incredibly slow, limiting its usefulness. Scientists at the National Energy Technology Laboratories (NETL) used the Cerebras CS-2 system, powered by the Cerebras Wafer-Scale Engine (WSE), in PSC’s Neocortex supercomputer to speed a classic fluid-flow simulation by several hundred times — fast enough to simulate the phenomenon as quickly as it happens in the real world.
WHY IT’S IMPORTANT
Fluid dynamics may be the most important natural phenomenon you haven’t thought much about. Whether it’s predictions of dangerous weather, design of cooling systems for electric power plants, protections against your mobile phone overheating, or whether the stuff at the back of your refrigerator is freezing, it all comes down to understanding how a fluid (which can be a gas, like air, or a liquid, like water) moves and flows.
The good news is that scientists know how to simulate fluid flow to a high degree of accuracy. The bad news is that, even with massive supercomputers, computational fluid dynamics (CFD) simulations are slow. They can help us understand what’s already happened. But they’re too slow and computationally expensive to help very much in predicting what’s going to happen, unless it’s months in advance.
“If you think about your commodity goods like refrigerators and stoves, as well as cars, planes and trains … and even the plastic goods that we buy, there’s always a process that’s involved that requires understanding how fluids move … The issue is that computational fluid dynamics falls in a class that’s incredibly data-intense … It’s not atypical for high-resolution CFD to take months to complete.”
— Dirk Van Essendelft, NETL
Dirk Van Essendelft, machine learning and data science engineer at the National Energy Technology Laboratories in Morgantown, W.V., working with scientists there and at Cerebras Systems as well as PSC’s Paola Buitrago, director of AI and Big Data, and her teammate Julian Uran, was looking for a way to speed his CFD software beyond what is possible even with the fastest traditional supercomputers. To achieve this the scientists turned to the Cerebras CS-2 system, deployed in PSC’s NSF-funded Neocortex supercomputer.
HOW PSC HELPED
By one way of thinking, Neocortex and its CS-2 systems were not an obvious choice for the project. Cerebras developed the CS-2, and PSC deployed it in Neocortex, to accelerate artificial intelligence “training,” which involves many rapid comparisons and inferences. On the other hand, CFD is a classic non-AI supercomputing application, involving sophisticated calculations for many, tiny portions of a fluid as it moves. But when Van Essendelft attended a Cerebras town hall event on the CS-2, he saw immediately how the technology could be the answer to the CFD problem.
The issue had less to do with computation than in moving data around inside the computer. The NETL scientists had access to a powerful “top 500” supercomputer on site, called Joule 2.0. With 74,240 CPU cores and powerful GPUs, the system ranks 47th in speed among supercomputers in the U.S., and 149th in the world. But the problem wasn’t processing speed — with connections between its processors that measured in tens of inches, and network connections that are measured in meters, like all traditional supercomputers, in a CFD simulation Joule took too much time for the processors to trade data and even access their own system memory.
When simulating the movement of hundreds of millions to billions of parcels of fluid, each computed by a separate processing core, it adds up to a data volume comparable to the entire world’s Internet traffic. Summing up all those moves, that means that the microseconds necessary to move a given bit of data in a conventional computer slows the computation greatly. That’s why even the most powerful supercomputers can take months to complete a CFD calculation.
Neocortex’s CS-2s, on the other hand, contains almost a million processing cores on each dinner-plate-sized WSE. Its connections measure less than a hundredth of an inch, with direct connections between neighboring cores. The original idea behind the WSE was to speed up an AI’s ability to trade data so that it could experiment with solutions as fast as possible. Van Essendelft saw that the same rapid exchange of data could also speed his CFD computations.
“We were able to [make] the … simulations much faster, which is a result of removing some of the traditional bottlenecks related to how fast I can access my own memory and how fast I can trade it with my neighbors … Paola [Buitrago] and her team were very instrumental in making sure that we could have the access we needed and helped work through all the technical issues [to avoid] lockups on the hardware — and that we were able to get data off the chip to create the visualization.” — Dirk Van Essendelft, NETL
In a proof-of-concept computation, Van Essendelft’s team applied Neocortex to the classic Rayleigh-Bénard convection problem, which shows how a fluid layer begins mixing as it’s heated from the bottom and cooled from the top in a gravitational field. Running the problem on one of Neocortex’s two CS-2s, they were able to complete the calculation with an expected speed gain of several hundred times. Cerebras and NETL announced the result and a resulting visualization video in February 2023. The team is still quantifying the exact speed gain and energy savings for this problem with the Swiss National Supercomputing Center. Previous work on similar problems has shown speed gains as high as 470 times.
The work is only just beginning. The scientists expect that, applied to other CFD problems, the Cerebras CS-2 can speed the computations in the range of 200- to 400-fold. That speed would enable real-time computation, in which the computer simulates the phenomenon as fast as it happens in real life. Such rapid predictions could enable CFD and its accuracy to be applied to a host of real-world problems, ranging from storm warnings to measuring uncertainty in designing new machines and systems to data-hungry but computationally similar simulations such as intruder detection and physical and cybersecurity.
The NETL work with Neocortex may also come full circle, back to AI. Van Essendelft sees a possible way of using AI running on the Cerebras CS-2 to do a “quick and dirty” simulation of the fluid flow, periodically checking it with a formal CFD calculation to ensure it’s still within the desired accuracy. He estimates that adding AI to the mix could speed the computations a further 10 to 100 times over and above the speedup already achieved.